AIOps, which stands for Artificial Intelligence for IT Operations, is here to stay. The truth is that leveraging artificial intelligence (AI) for ITOps offers a range of benefits that can significantly improve the efficiency, reliability, and performance of IT operations.
So keep on reading as we explore AIOps software potential. From automating routine tasks to predicting future issues and enhancing decision-making, as well as practical scenarios as strategies for its implementation.
Let’s get into it.
Table of contents
- What is AIOps?
- Why is AIOps important?
- 10 benefits of using artificial intelligence for IT operations
- How does AIOps work?
- How to develop an AIOps strategy
- 5 AIOps examples
- 8 common features for AIOps tools
What is AIOps?
AIOps is a multi-layered technological platform that makes use of machine learning (ML) and analytics to automate IT operations.
In a nutshell, AIOps uses big data to gather a range of information from different IT operations tools and devices so that problems can be identified automatically and addressed in real time.
This method offers insights that were previously hard to find or needed a lot of human labor to achieve, which helps to improve the efficiency, performance, and dependability of IT processes.
AIOps vs. DevOps
While AIOps and DevOps both aim to improve IT operations, they focus on different aspects and employ distinct approaches.
In terms of their focus, DevOps aim is to increase an organization's ability to deliver applications and services at high velocity, with an emphasis in collaboration between development and operations teams. AIOps, on the other hand, focuses on applying artificial intelligence and machine learning to automate and enhance IT operations.
The way they operate is also different. You have DevOps which relies on automation tools that facilitate the software development lifecycle with continuous integration (CI), continuous delivery (CD), Configuration Management, and version control. AIOps uses big data analytics, AI, and ML algorithms to analyze data from various IT operations tools and devices, so they can predict and prevent potential issues before they impact the business.
And because their approaches are poles apart, their outcome is too. DevOps reduces the length of the development lifecycle, encourages teamwork, and raises the caliber of software releases. AIOps aims to identify possible system problems, automate IT operations activities, and deliver useful information to stop downtime or performance degradation.
And lastly, when it’s time for implementation DevOps practices are implemented throughout the software development lifecycle, influencing how teams collaborate, how software is built, tested, and deployed. AIOps is applied across IT operations, focusing on automating and optimizing operational tasks, monitoring, and incident response.
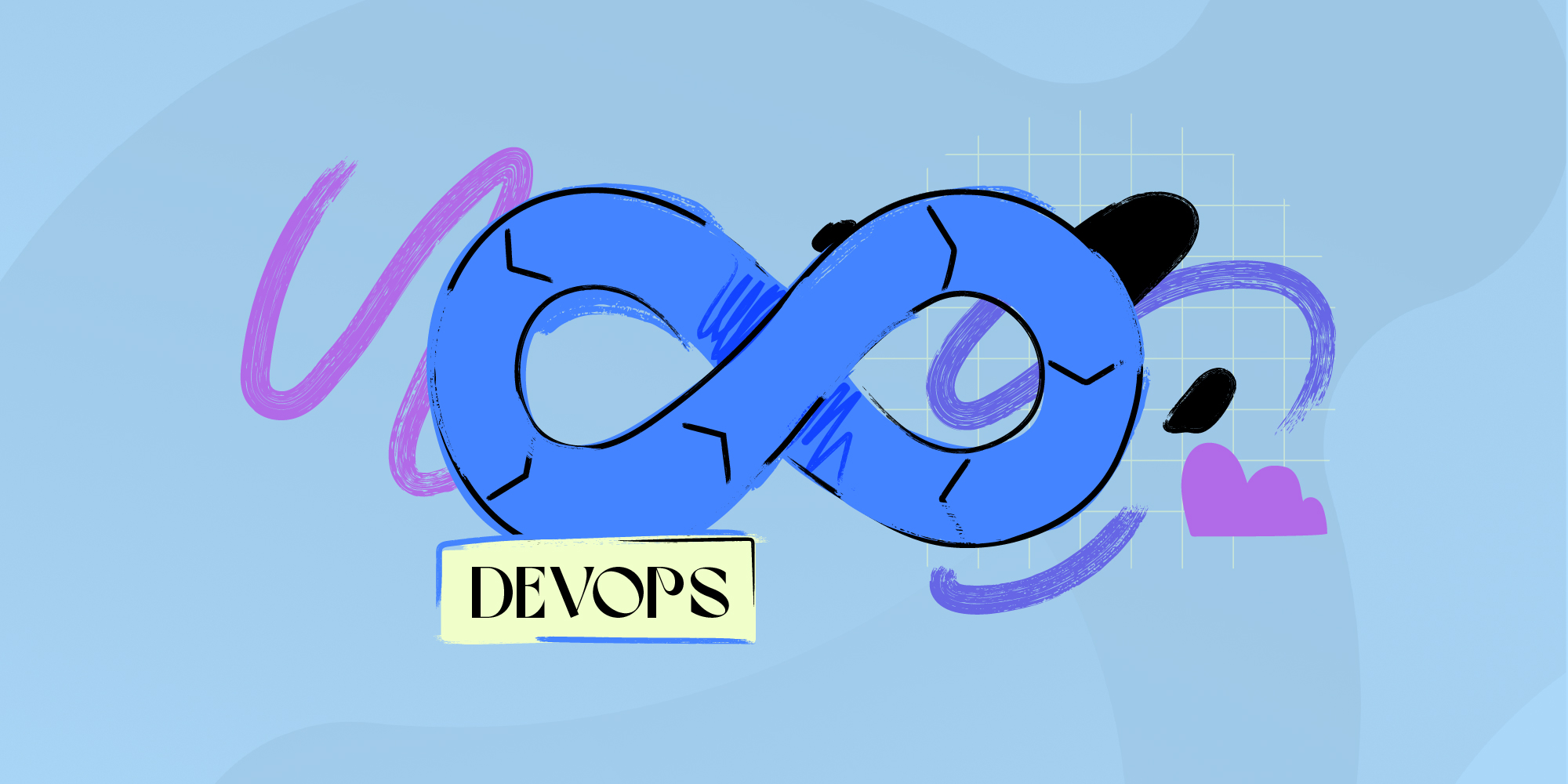
DevOps Explained
Why is AIOps important?
AIOps is important for several reasons, primarily due to its impact on the overall performance of an organization's IT infrastructure.
One of its best capabilities is that it saves time and reduces the potential for human error.
This is because AIOps automatically detects and resolves issues in real-time, which reduces downtime and the need for manual intervention. We could also say that when it can leverage ML and AI, it is easier for AIOps to predict potential problems before they occur, allowing IT teams to proactively address them.
As a result, IT teams enhance their performance monitoring since AIOPs offers an unified view of all operations to identify and rectify inefficiencies more effectively. As its nature is data-driven, one would see trends, forecast needs, and allocate resources better.
Now, why should companies care about AIOps apart from giving them the competitive edge that is crucial in today's fast-paced market? Because it needs less IT resources and could potentially lower investment in reactive measures. And, we all know that in this digital world downtime leads to significant losses, not only in money, but also in loyalty. Customer experiences are often directly tied to the performance of IT services.
As businesses grow, their IT infrastructure becomes more complex. AIOps seems to manage this complexity by automating operations and providing support during scalability efforts and when adapting to new technologies to stay ahead of the curve.
Ultimately, you want to free up your IT teams’ time to focus on strategic initiatives rather than firefighting.
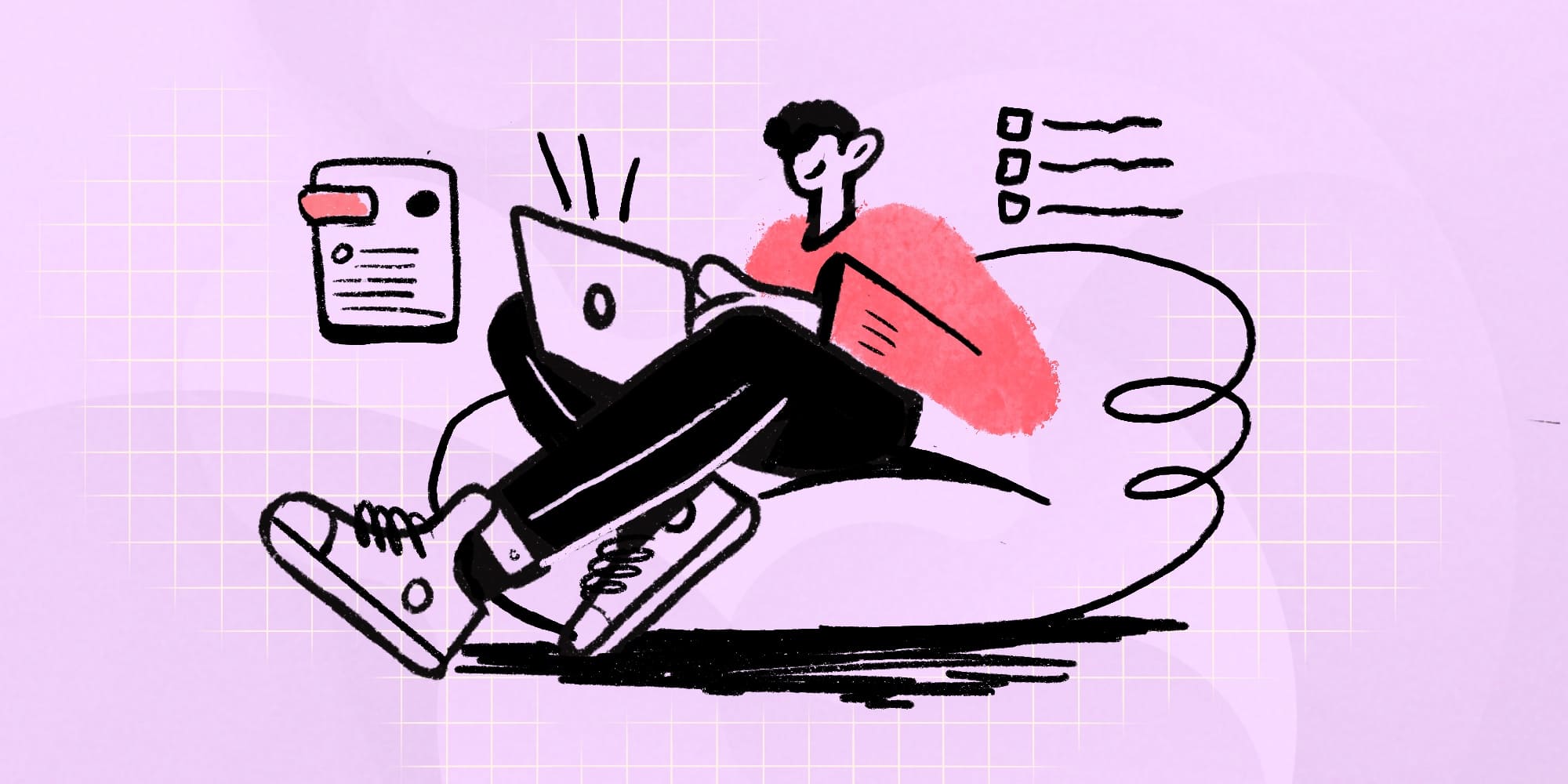
9 Ways to Do Proactive IT Support – And Switch From The Break/Fix Model
10 benefits using artificial intelligence for IT Operations
The integration of AI into ITOps provides several key benefits that can significantly enhance operational efficiency, reliability, and performance. Considering its automation and pattern recognition capabilities.
Here are the main advantages of using AI for IT Operations.
1. Enhanced operational efficiency
AI automates routine and repetitive tasks, reducing the workload on IT staff and allowing them to focus on more strategic initiatives. So, with automation teams can focus on improving the company's IT infrastructure.
2. Predictive analytics
The predictive capability of AI allows proactive measures to prevent downtime or potential issues before they impact services. Consider a situation where an AI system analyzes patterns in server performance data and predicts a potential overload during peak times. If that were the case the IT team could adjust resources to prevent a server crash.
3. Faster incident resolution
This one goes in line with the previous point, since AI can quickly analyze incidents, identify their root causes, and suggest or even automate corrective actions. For example, when a network outage occurs, AI quickly sifts through data to pinpoint that a specific router malfunctioned. It either restarts the router automatically or alerts the IT team.
4. Improved decision-making
IT leaders can use it to optimize resource allocation, plan capacity, and make strategic investments in IT infrastructure. This is because AI analyzes past data on IT resource usage during product launches.
5. Enhanced user experience
AI-driven ITOps ensures that IT services are running smoothly and efficiently, so businesses can offer a better experience to their customers and employees. In practice, AI monitors an application's performance in real-time. It notices a slowdown and automatically reallocates resources to improve speed, thus maintaining a seamless user experience without any manual intervention.
6. Cost reduction
Automating IT operations with AI can lead to significant cost savings. Pretty much because you reduce the need for manual intervention. For instance, if a company were to automate the monitoring of cloud resource usage, AI would identify and shut down underutilized instances, cutting unnecessary costs and optimizing cloud spend.
7. Scalability
As we mentioned before, AI can easily scale to meet the growing complexity and volume of IT operations. In fact, AI systems automatically scale up resources during high demand and scale down when demand decreases. This scalability ensures IT infrastructure growth and the deployment of efficient resources, without compromising on performance or reliability.
8. Security enhancement
This one is a no-brainer: IT security. It goes hand in hand with its monitoring capabilities for pattern recognition. AI continuously scans for unusual network traffic patterns, quickly identifying a potential cyber attack. It either blocks the threat automatically or alerts the security team, minimizing potential damage.
9. Continuous improvement
AI systems learn from data and foster continuous improvement. It’s a learning capability that allows AIOps to adapt to new challenges and technologies. This is the case because an AI system learns from each incident to improve its accuracy in predicting and responding to future issues. Over time, it becomes more adept at managing the IT environment, reducing errors and improving efficiency.
10. Cross-domain insights
AI can analyze data across different domains and silos within an IT environment to enable better coordination and optimization of IT resources across the entire organization. To illustrate it: AI could analyze data from both the company's website and internal applications, identifying a correlation between increased website traffic and internal system load. Then, the outcome would be to prepare the infrastructure for future traffic surges.
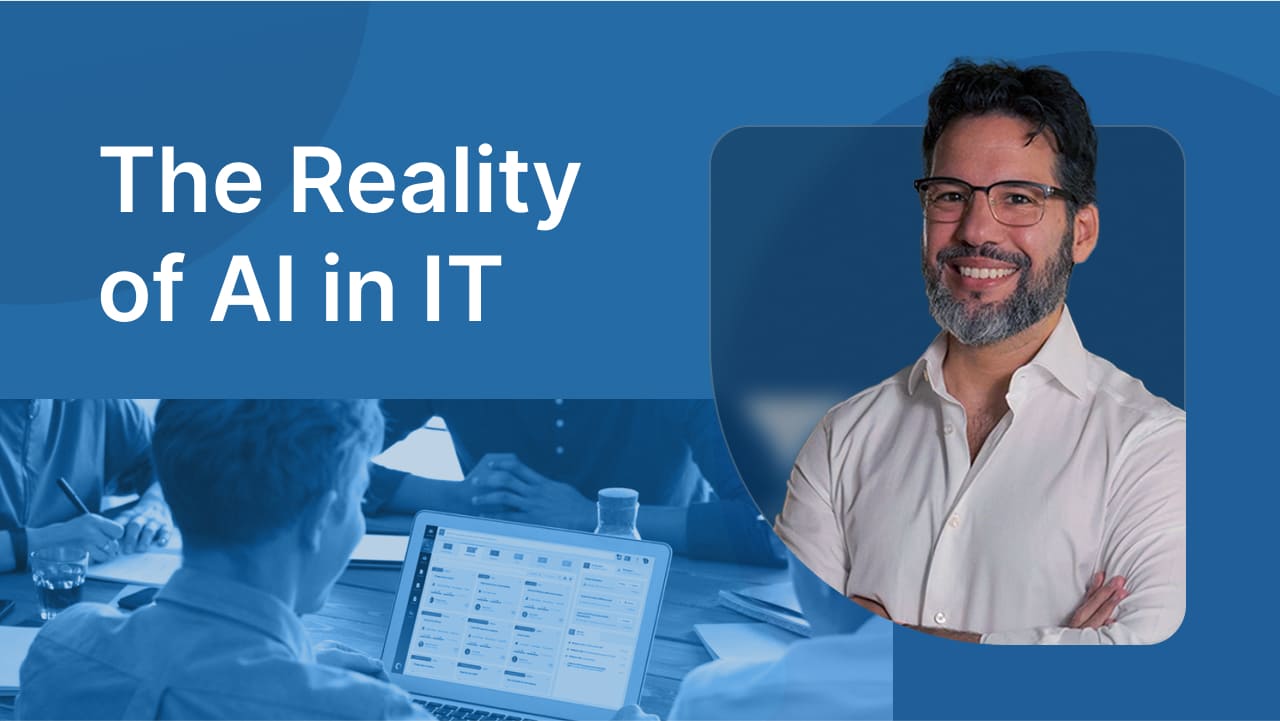
Bridging The Gap: The Marketing Hype vs. The Reality of AI in IT
How does AIOps work?
In simpler terms, AIOps works by gathering and analyzing massive amounts of data from diverse IT operations sources, and then applying AI to find patterns, predict potential problems, and automate solutions.
Its core elements are:
- Machine Learning
- Performance baselining
- Anomaly detection
- Automated root cause analysis
- Predictive insights
But let’s break it down into steps.
1. Data collection
The first step in the AIOps process is to gather data from various sources within the IT environment. This data can include logs, metrics, performance data, and incident reports from servers, databases, applications, and networking equipment. AIOps platforms aggregate this data into a centralized system, making it accessible for analysis.
2. Data processing and analysis
Once data is collected, it undergoes processing to structure and normalize it for analysis. AIOps uses machine learning algorithms and statistical models to analyze this vast amount of data in real-time. The analysis aims to identify patterns, anomalies, and correlations that might not be evident to human operators.
3. Pattern recognition and anomaly detection
AIOps learns from historical data to identify what constitutes normal behavior for the system. It can then detect deviations from this norm, which may indicate potential issues or performance bottlenecks. Early detection of anomalies allows IT teams to address problems before they impact users.
4. Predictive analysis
AIOps predicts which components might fail and when, allowing for proactive maintenance and preventing downtime. This is great for planning and optimizing IT operations.
5. Automation and remediation
Perhaps the most impactful aspect of AIOps is its ability to automate responses to identified issues. Based on the analysis, AIOps can trigger automated workflows to remediate problems without human intervention. For example, it can automatically restart a failed service, scale resources to meet demand, or reroute traffic to prevent congestion. Automation speeds up response times and reduces the manual workload on IT staff.
6. Continuous learning
AIOps platforms are designed to learn and improve over time. They adapt their models based on new data and outcomes, becoming more accurate and efficient in their predictions and recommendations.
How to implement AIOps? Developing an AIOps strategy
Developing an AIOps plan is a forward-thinking decision that aligns with the changing landscape of IT operations.
The novelty of AIOps presents both opportunities and challenges. On one hand, it offers the potential to significantly improve efficiency, reduce downtime, and predict issues before they impact operations. On the other hand, its newness means that best practices are still being established, and there is a learning curve associated with its adoption.
We all agree that its implementation not only takes time, but it requires a mindset shift.
Hence, this is the best way to go about it:
Engaging stakeholders
For AIOps to truly make an impact, it's essential to ensure it aligns with your organization's overarching goals. Engage all key stakeholders — this includes IT personnel, management, and end-users — to make sure they're on board and fully understand the benefits and changes that AIOps will introduce. Given that AIOps is a relatively new field, it is vital to communicate about its potential and how it will be integrated into existing workflows.
Selecting the right tools
Finding the appropriate AIOps platform for your organization requires careful consideration. The platform should not only match the scale and complexity of your operations but also be capable of integrating seamlessly with your existing systems.
Choose a tool that can aggregate event data across various systems, applications, and infrastructure components. Additionally, take stock of your current tools and skill sets to identify any gaps that need to be addressed to effectively implement and utilize AIOps technologies.
Developing necessary skills
Implementing AIOps successfully demands a unique combination of IT operations expertise and data science knowledge. Make sure your team is equipped with the required skills by providing access to training and education. If necessary, consider collaborating with external experts who can bring in-depth knowledge of AIOps to your organization.
Implementing in phases
Given the novel nature of AIOps, adopting a phased approach to implementation can be a prudent strategy. Start with systems that are critical but not mission-critical to mitigate risks and give your team the opportunity to build their expertise with the AIOps platform. This gradual rollout allows for adjustments and learning, ensuring a smoother transition to more extensive AIOps adoption across your IT environment.
5 AIOps examples to put into practice
Now that we have explored what AIOps is and how to develop a robust implementation strategy, let’s take a look at some examples of what this looks like in practice.
1. Fraud detection in financial services
Think of the millions of transactions that a bank processes daily and how fast their system has to be to detect fraud. To prevent financial loss and maintain their customer’s trust an AIOps system would analyze transaction patterns in real-time. With automation it can flag suspicious activity immediately and block them for further verification.
2. Optimization of retail supply chain
In this scenario, IAOps predicting capabilities can plan distribution strategies such as recommending adjustments to orders in case it detects low inventory in the stock levels across a store or a chain of them.
3. Equipment maintenance at manufactures
Imagine how manufacturing plants that rely on heavy machinery for regular maintenance could benefit from AIOps to prevent unexpected breakdowns and costly downtime. These businesses can use the technology to schedule this automatically, while also using it to analyze data from equipment sensors to predict when machines are likely to fail or require maintenance.
4. Network performance in telecommunication companies
A telecom company could implement AIOps for continuous monitoring and analysis of network traffic and performance metrics. The system can identify potential network issues and predict outages. As a result, it could initiate automated responses to mitigate these issues before they impact customers.
5. Its possible uses in the healthcare system
Medical monitoring using AI is still being researched as ethical and legal considerations take longer to assess. Nonetheless, in the future we can expect, for example, hospitals to use AIOps to aggregate and analyze data from various monitoring devices with the hope of predicting potential health issues before they become critical, alerting medical staff to intervene promptly.
As of now, CP24 reported that the largest consortium of research hospitals in Canada has named a chief artificial intelligence scientist Bo Wang, to leverage cutting-edge technology capable of accelerating diagnostic processes, enhancing and tailoring patient treatment, and reducing recovery periods
The idea behind this is to unite physicians and researchers engaged in AI applications across various domains, such as oncology and heart disease.
8 common features for AIOps tools
While the specific features of AIOps tools can vary depending on the product, when choosing THE one for your IT team we think there are key features you can’t compromise not having as these go in concordance with the benefits we mentioned above.
- Data aggregation and normalization of this data to create a unified data model that runs analyses and finds correlations from different systems.
- Anomaly detection to help IT teams to detect issues early, often before they impact users.
- Event correlation and analysis is often ignored, but it’s great for correlating and filtering disparate events and logs, helping to pinpoint the root cause of issues.
- It goes without saying that one of the most useful features is their predictive capability, which allows IT teams to proactively address problems before they occur, improving system reliability and performance.
- Automated going hand in hand with remediation, meaning that the AIOps automation not only speeds up the resolution process but also frees up IT staff to focus on more strategic tasks.
- The dashboard and visualization needs to be comprehensive and provide real-time insights into IT operations. These visual tools help IT teams to quickly understand the state of the IT environment.
- To function effectively within an IT ecosystem, AIOps tools need to have integration capabilities with other IT management and monitoring tools so all actions become part of an automated workflow.
- And last but not least, AIOps tools must handle increasing volumes of data and more complex operations. Hence, scalability ensures that your business can grow and evolve.
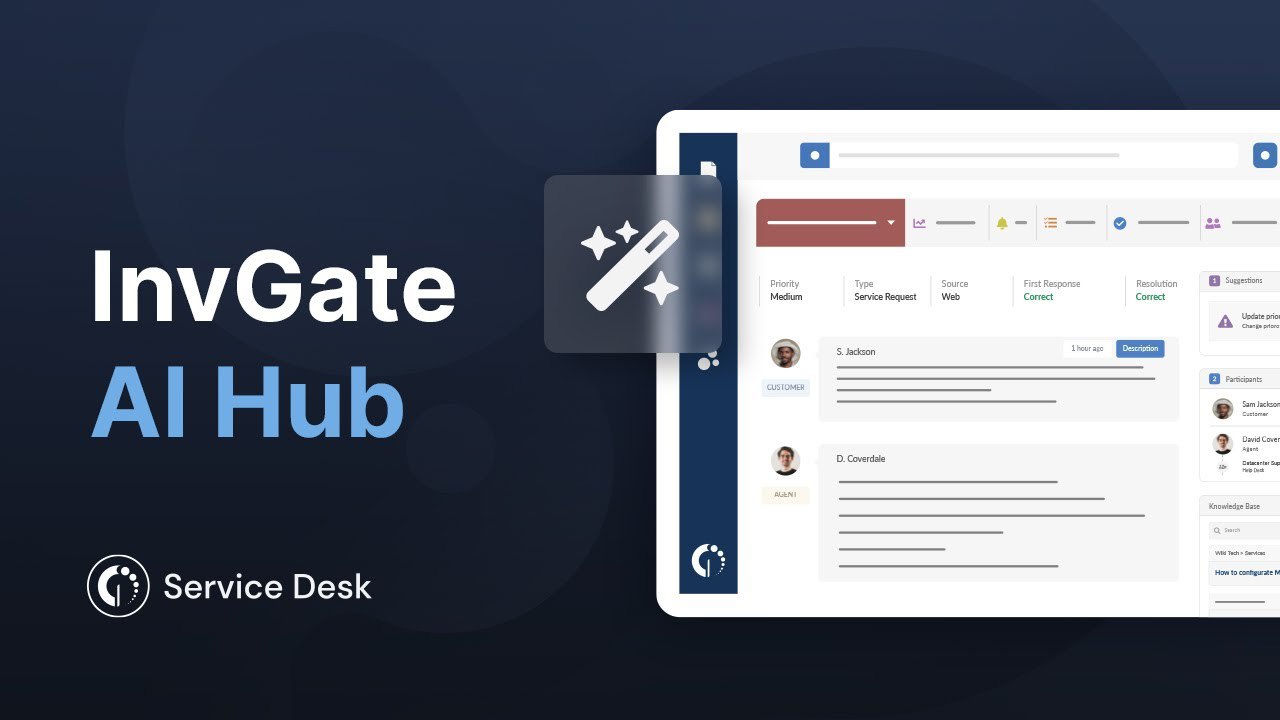
InvGate AI Hub: New Artificial Intelligence Capabilities For IT Teams
In conclusion
To wrap up, AIOps detects, predicts, and mitigates IT incidents. As a tool all its features collectively transform IT operations by making them more proactive, efficient, and responsive to the needs of your business.
Many examples and scenarios illustrate the versatility of AIOps across different industries, but also its operational challenges. That being said, there is no denying that its potential to drive significant improvements in every business makes this technology unstoppable.
Frequently Asked Questions
What does AIOps stand for?
AIOps stands for Artificial Intelligence for IT Operations. It refers to the application of artificial intelligence (AI), including machine learning and big data analytics, to enhance and automate IT operations. AIOps platforms are designed to analyze the massive amounts of data generated by IT systems and services, identify patterns and anomalies, and provide actionable insights to prevent or resolve issues in real-time.
Who coined the AIOps term?
The term "AIOps" was coined by Gartner, a leading research and advisory company. The concept was born out of the need to manage the increasing complexity and scale of IT environments and data, driven by the rise of Artifitial Inteligence (AI) and the adoption of cloud services, microservices architectures, and other advanced technologies.
What is observability in AIOps?
Observability in AIOps refers to the capability to gather, analyze, and act on data from across an IT environment to gain insights into system performance and health. It enables organizations to detect and diagnose issues more effectively, often before they impact users, by providing a comprehensive view of the IT infrastructure, applications, and services.
How to learn AIOps?
To learn AIOps you need to combine theoretical knowledge with practical experience and ongoing learning:
- Begin with learning the basics of IT operations, including system administration, network management, and cloud services.
- Familiarize yourself with key concepts in AI and machine learning.
- Experiment with AIOps tools and platforms. Many vendors offer free trials or community editions of their software.
- Engage with AIOps communities online.
- Stay up to date by reading industry publications and attending conferences or workshops related to AI and IT operations.