You've probably heard a lot about artificial intelligence (AI) lately—from chatbots to self-driving cars: AI seems to be everywhere. But there's one more development you might not be familiar with yet: multimodal AI.
While traditional AI systems focus on a single type of data, such as text or images, multimodal AI takes it further by processing multiple types of data at the same time.
So, what makes this type of AI different, and why is it so important? Well, we'll tell you this in advance: Multimodal AI has the potential to transform how machines understand and respond to the world around them.
Let’s explore multimodal AI, how it works, and the real-world applications it’s already starting to influence.
What is multimodal AI?
Artificial Intelligence (AI) has evolved rapidly, branching out into areas like natural language processing (NLP), computer vision, and speech recognition. Multimodal AI takes this development a step further by integrating multiple forms of data—text, images, video, and even audio—into one model. Rather than focusing on a single type of input, such as only text or only images, multimodal AI can analyze and combine these various data types to deliver more accurate, nuanced results.
For example, when interacting with a voice assistant, multimodal AI could interpret both spoken commands (audio) and visual cues from a connected camera (video) to provide a better response. It’s not just about understanding one form of data; it’s about integrating them to get a fuller, more detailed picture of a situation.
How multimodal AI works
Multimodal AI operates by combining different types of data inputs (modalities) and then processing them together. This is done using advanced machine learning models like deep learning networks. Here's a breakdown of the process:
1. Data processing by modality
Each type of data is processed individually at first. For instance, text might be analyzed using NLP techniques, while images are processed using computer vision algorithms such as Convolutional Neural Networks (CNNs).
2. Fusion of modalities
Once each data type has been analyzed, the outputs are fused. This fusion allows the AI model to integrate the different inputs, leading to a more comprehensive understanding. For example, in a self-driving car, video from cameras, radar data, and spoken instructions can all be combined to inform the car’s decisions.
3. Decision making
After the data has been fused, the AI makes decisions based on the combined information. This could mean generating a response to a query, offering a recommendation, or triggering an action—such as stopping a self-driving car if an obstacle is detected both by the camera and the radar.
This fusion of modalities is what sets multimodal AI apart from traditional AI models. By bringing together different types of data, it can make more informed decisions that would be difficult or impossible with just one type of input.
Why is multimodal AI important?
Multimodal AI is important because it mirrors the way humans perceive and process the world. We don’t rely solely on what we see, hear, or read—we integrate all these forms of input to make decisions. AI that can do the same opens up new possibilities across various fields.
Some of the key advantages of multimodal AI include:
- Greater accuracy: By processing more than one type of data, multimodal AI can reduce errors and improve its performance.
- Richer context: Different data types provide different kinds of information. Combining them offers a fuller, more detailed understanding of a situation.
- Enhanced versatility: Multimodal AI can be applied in a wide range of scenarios, from medical diagnostics to virtual assistants, offering businesses and organizations more flexibility.
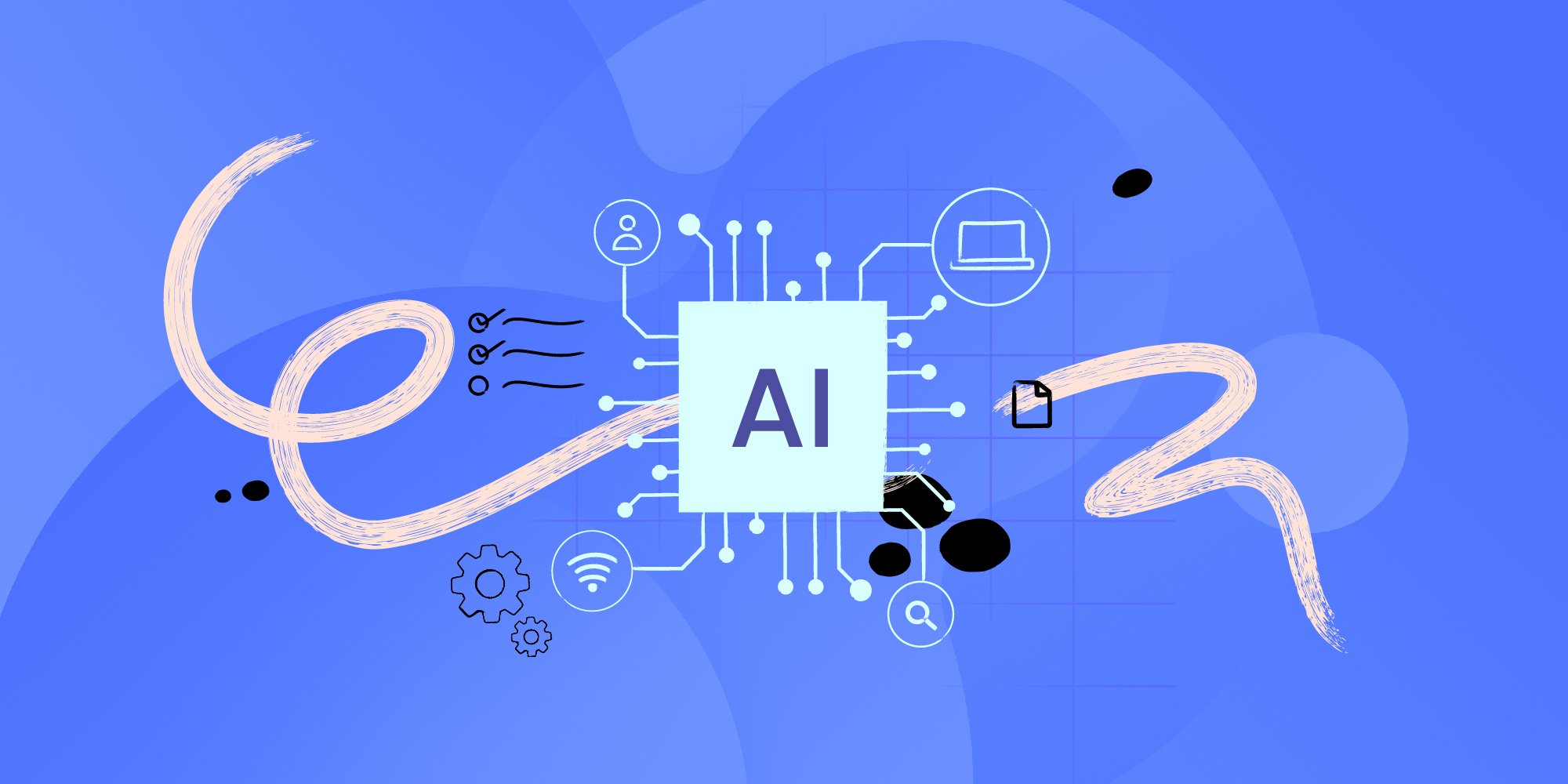
92% of The Largest Tech Companies Worldwide Have an Ongoing AI Project
Key real-world applications of multimodal AI
1. Healthcare
In healthcare, multimodal AI is transforming how patient data is analyzed. By combining medical records (text), diagnostic images (such as MRI scans), and even speech data from doctor-patient consultations, AI can offer more personalized treatment recommendations and better diagnoses.
For instance, a multimodal AI system might analyze both a CT scan and a patient’s symptoms described in medical notes to identify the likelihood of a particular disease. This combined approach leads to faster, more accurate diagnoses, reducing the chances of misdiagnosis or delayed treatment.
2. Autonomous vehicles
Autonomous vehicles rely heavily on multimodal AI. These vehicles need to process multiple forms of input simultaneously, such as video from cameras, radar data, and GPS signals. Multimodal AI helps these systems make real-time decisions—like recognizing a pedestrian in the crosswalk, even when it’s difficult to see due to poor lighting conditions.
By integrating video footage with other sensor data, the car can make better-informed decisions than it could by relying on just one input, such as cameras alone.
3. Content moderation
Social media platforms face the challenge of moderating vast amounts of content across multiple media types—text, images, videos, and more. Multimodal AI is increasingly used to help moderate this content efficiently and fairly.
For example, detecting inappropriate behavior or hate speech often requires analyzing both the text of a post and the images or videos associated with it. Multimodal AI can cross-reference these different inputs to identify harmful content more effectively.
4. Virtual assistants and Customer Support
Multimodal AI enables virtual assistants, like Siri or Alexa, to become more responsive and capable. These assistants can provide more accurate responses by combining voice recognition (audio) with other inputs, such as text or images.
In customer support, multimodal AI can enhance chatbot interactions by analyzing both the tone of a customer’s voice (audio) and their text inputs. A chatbot could detect frustration in a customer’s voice and prioritize more urgent support.
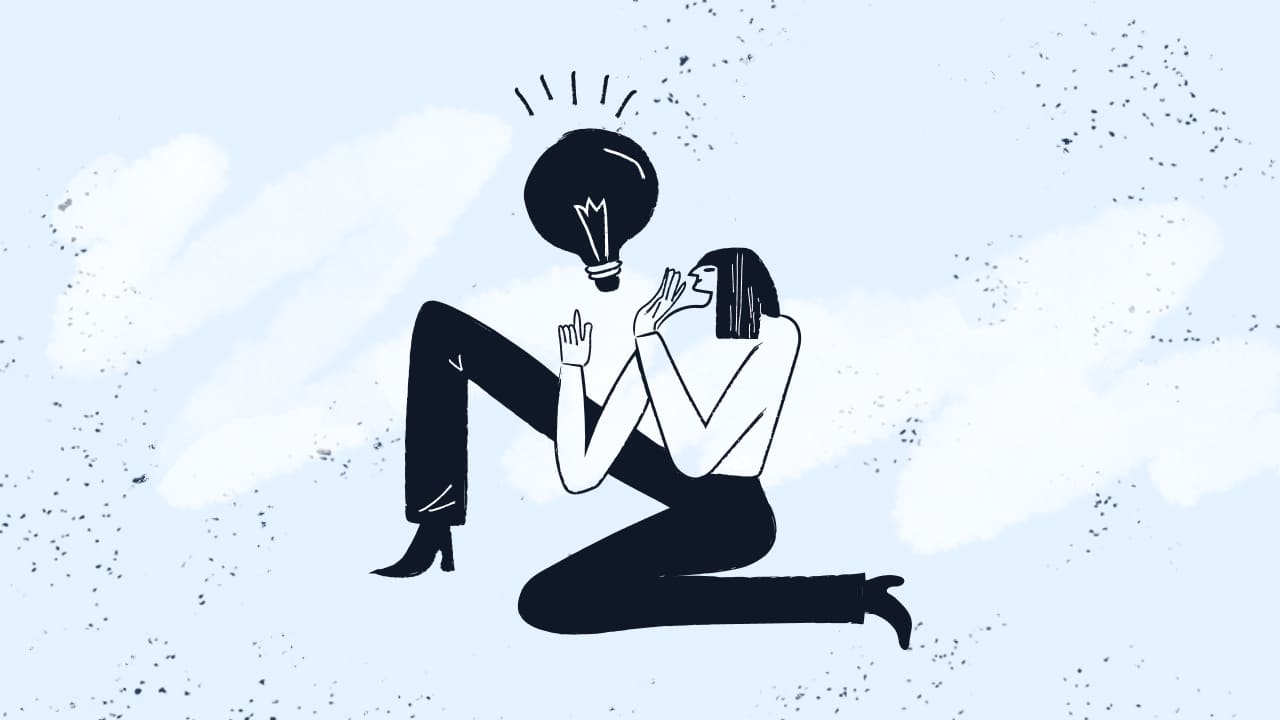
AI + Service Desk: 7 Ways to Boost Service Desk Capabilities
How multimodal AI enhances human-AI interaction
One of the most significant benefits of multimodal AI is how it enhances interaction between humans and machines. Traditional AI models often fail to understand context or handle ambiguous inputs because they rely on a single data type. Multimodal AI, on the other hand, brings more depth to human-AI interactions by analyzing different inputs together.
In a conversation, for example, a person’s tone of voice can drastically change the meaning of the words they say. Multimodal AI can recognize these subtleties. By analyzing both text and audio, AI can understand when a user is being sarcastic or when they are serious, providing more meaningful responses.
Multimodal AI also opens up opportunities for improving accessibility. For instance, people with visual impairments could benefit from AI that uses speech and audio to assist in navigating websites or reading documents. Similarly, those with hearing difficulties could rely on AI that integrates text and visual cues to enhance communication.
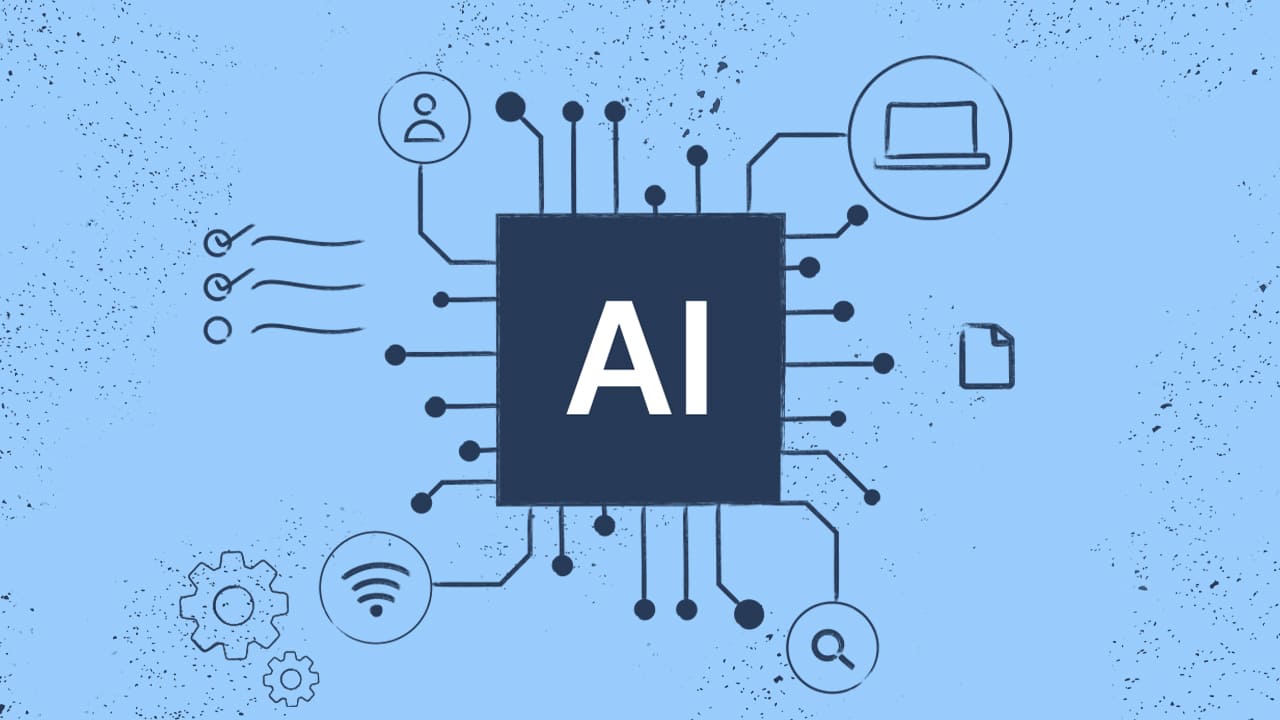
5 Best AI IT Support Tools in 2024
Challenges of multimodal AI
While multimodal AI holds great promise, it’s not without its challenges. Here are a few of the primary obstacles developers and businesses may encounter:
1. Data integration
One of the most significant hurdles in implementing multimodal AI is managing the integration of different data types. Text, images, and video have distinct structures, and processing them together can be complicated. Each data type requires different models and algorithms for analysis, and combining these outputs in a meaningful way can be difficult.
2. Complexity of models
Multimodal AI models are more complex than single-modality models. Training these models often requires more computational resources and time, and debugging errors can be challenging. Additionally, ensuring that each modality is equally weighted and contributes effectively to the final decision-making process requires ongoing optimization.
3. Data availability
For multimodal AI to function properly, large datasets that span different modalities are needed. Accessing high-quality data from multiple sources (e.g., text, images, and videos) can be difficult and costly, especially in industries that are new to AI applications.
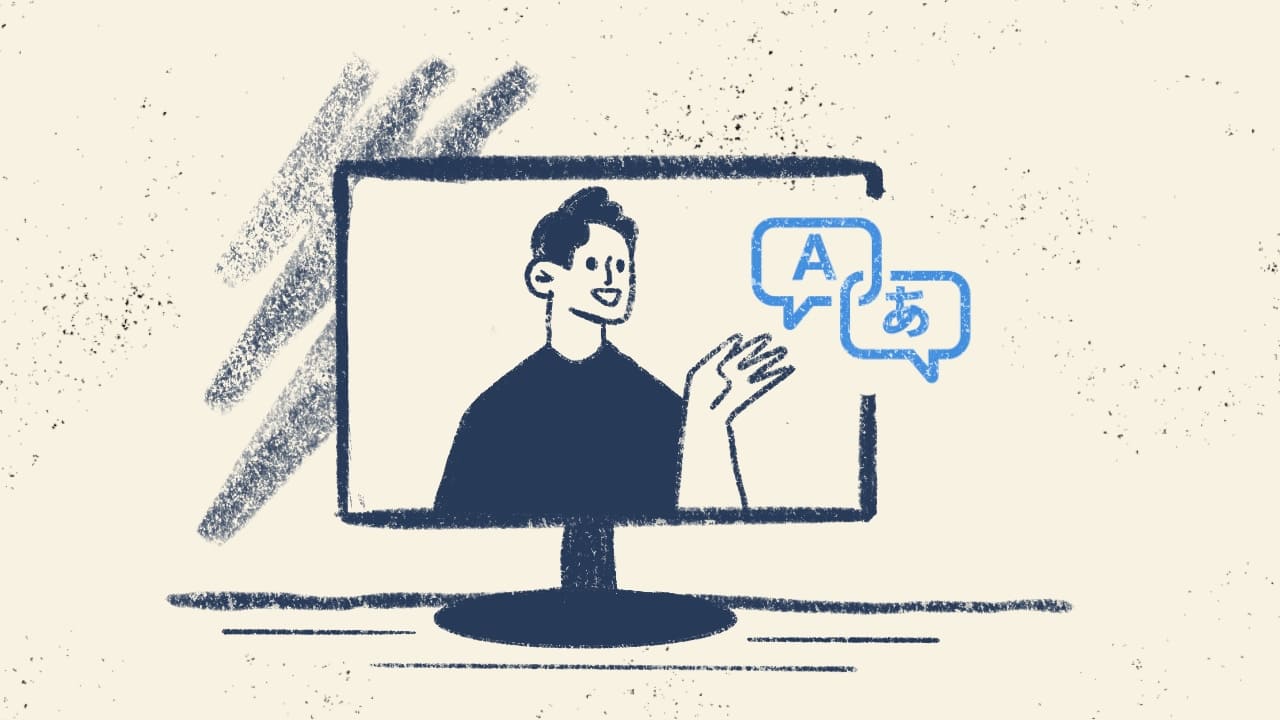
What is Data Annotation? Its impact on AI development.
4. Real-world implementation challenges
While multimodal AI holds great potential, implementing it in real-world systems is still a challenge. One major issue is the synchronization of data. In applications like autonomous vehicles or robotics, the AI system must process data from multiple sensors in real-time, and any lag in one modality could lead to errors or poor decisions.
Additionally, ensuring that the model remains robust when one data source is temporarily unavailable (e.g., a camera malfunction in a self-driving car) is another hurdle researchers are working to overcome.
Finally, there are ethical and privacy concerns surrounding multimodal AI, especially in applications that involve surveillance or content moderation. When multiple forms of data are combined, it increases the potential for bias or misuse. Developers need to consider how to implement these models responsibly and transparently to avoid unintended consequences.
Who is developing multimodal AI?
The development of multimodal AI is being driven by some of the biggest players in technology, as well as academic institutions and specialized AI research labs. Companies like OpenAI, Google DeepMind, Microsoft, and Meta (formerly Facebook) are leading the charge, investing heavily in this technology to push the boundaries of what AI can do.
These organizations are building complex models that combine various machine learning techniques, like neural networks, computer vision, and natural language processing (NLP), to create systems that can process and understand multiple types of data simultaneously.
OpenAI and GPT models
OpenAI, known for its language model GPT-4, is also contributing to multimodal AI development. While GPT-4 itself focuses on text-based tasks, OpenAI has been working on models that extend beyond text. In fact, OpenAI introduced DALL-E, a multimodal model that can generate images based on textual descriptions.
This is a prime example of how AI can integrate different data types (in this case, text and images) to perform tasks that would be challenging for single-modality models.
Google DeepMind’s multimodal research
Google DeepMind has long been a leader in AI research, and multimodal AI is no exception. DeepMind’s Perceiver model is designed to handle multiple types of data, such as images, audio, and video, making it highly versatile. The Perceiver uses a unified architecture to process different modalities, which helps overcome some of the challenges of data integration that traditional models face.
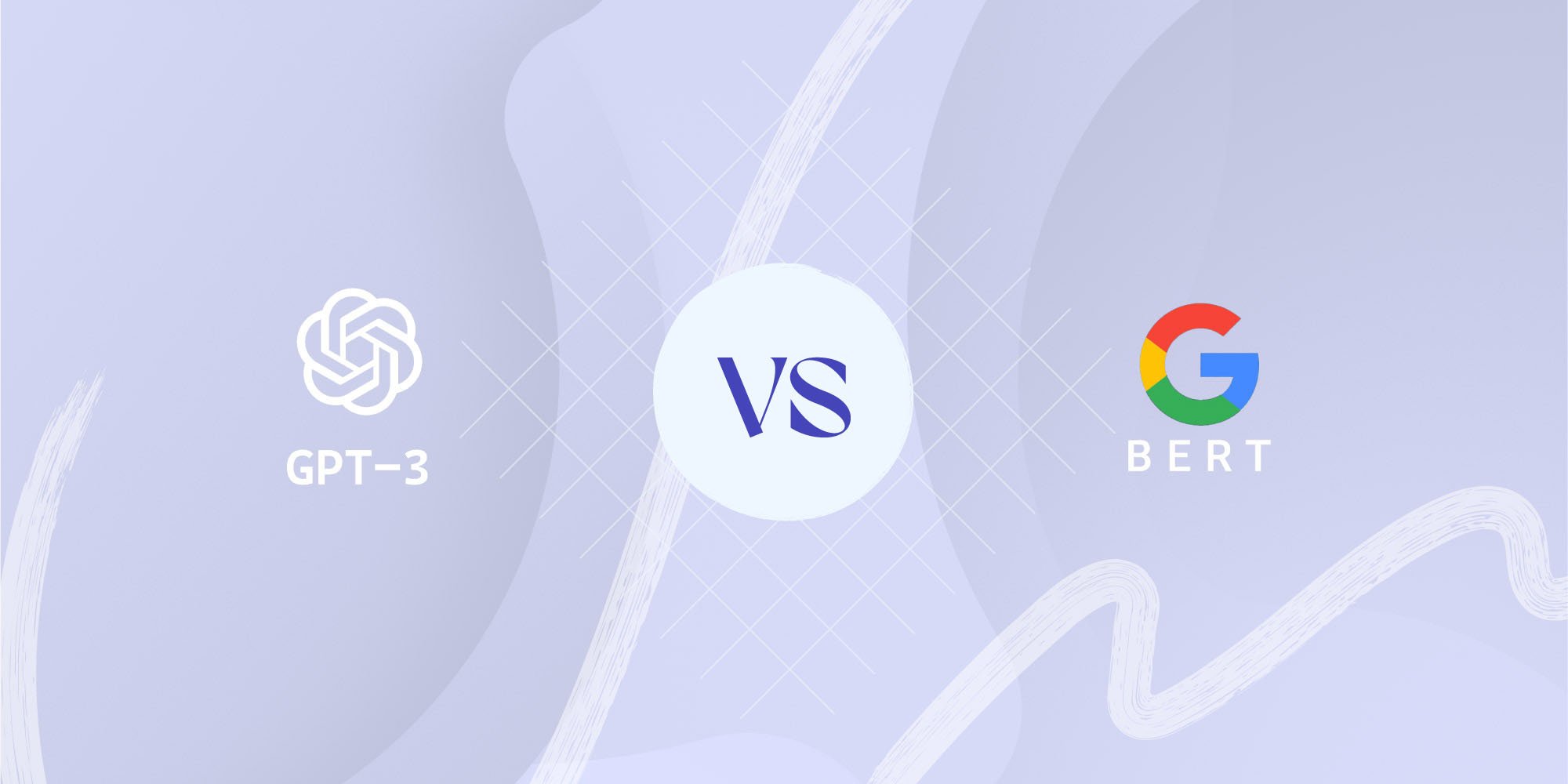
BERT vs GPT: Comparing the Two Most Popular Language Models
Microsoft and multimodal fusion in Azure AI
Microsoft has integrated multimodal AI into its cloud-based Azure AI services, allowing businesses to leverage this technology for real-world applications. Microsoft’s Turing-NLG model and CLIP (Contrastive Language–Image Pre-training), a joint project with OpenAI, are examples of multimodal systems that fuse text and images.
The company is focused on making multimodal AI more accessible to industries, allowing organizations to build smarter, more responsive systems for tasks like customer service, predictive maintenance, and business analytics.
Meta’s Multimodal AI Initiatives
Meta has also entered the multimodal AI space with projects like ImageBind, which links data from different modalities—images, text, and audio—to create AI systems that understand and interact with the world more like humans do.
Meta is developing AI that could eventually power more immersive virtual environments, better content moderation on social media platforms, and more advanced virtual assistants.
Conclusion
Multimodal AI represents a significant step forward in artificial intelligence by enabling systems to process and integrate various types of data. Its applications are far-reaching, from healthcare and autonomous vehicles to content moderation and customer service. As AI systems continue to advance, the ability to handle multimodal inputs will be essential for creating more accurate, responsive, and intelligent systems.
Understanding multimodal AI's basics and challenges will help businesses, developers, and organizations make better decisions about its adoption and integration. With the right strategy and tools, multimodal AI has the potential to revolutionize how we interact with technology, offering richer and more versatile capabilities than ever before.