We celebrate “disruptiveness” in the tech space for its potential to revolutionize industries and drive innovation, often focusing on the excitement of what's new. But true disruption doesn't just add to our toolkit; it challenges us to rethink the very foundations we've relied on, including how to measure success.
Organizations use metrics like productivity, efficiency, and user satisfaction to gauge how new technologies are performing and to steer their decisions. These measurements are the lifeblood of continuous improvement and strategic planning. But as disruptive technologies emerge, the metrics we’ve relied on for decades can suddenly become inadequate or even misleading.
Artificial intelligence, with its profound ability to transform processes, is one such disruptive force. By automating tasks that once required human intervention, AI changes how we measure success.
Traditional metrics were developed in a world where human agents handled all requests. But as AI takes over more straightforward tasks, resolving issues before they even become tickets, these metrics no longer paint an accurate picture of an organization’s performance. Instead, they risk obscuring the true value that AI brings to the table.
The limitations of traditional metrics
For years, metrics like mean time to resolution (MTTR) have been the bedrock of IT Service Management, providing a clear, quantifiable measure of how efficiently a team handles issues.
These metrics were designed for a world where human agents were responsible for resolving tickets, each step of the process meticulously tracked – from the moment a request was made, through ticket creation, assignment, and finally, resolution. In this context, MTTR offered a reliable snapshot of performance, guiding improvements and helping organizations meet their service level agreements.
However, the landscape has shifted dramatically with the advent of AI. When an AI agent steps into the picture, the traditional playbook no longer applies. AI has the capability to resolve issues before they even enter the system as a ticket, completely bypassing the conventional metrics we've depended on.
This is where the cracks in our old systems start to show. As AI takes on more of the routine tasks, the tickets that do get created are often the complex, thorny issues that AI can’t handle – at least, not yet.
This shift leads to an apparent increase in resolution times, but this doesn't mean that efficiency is dropping. On the contrary, it indicates that AI is effectively handling the simpler issues, leaving only the more challenging cases for human agents.
In this new reality, relying solely on traditional metrics not only risks misinterpreting the effectiveness of your IT team but also overlooks the significant contribution AI is making by quietly resolving issues in the background. This calls for a fundamental reassessment of how we measure success in an AI-enhanced environment.
The need for new metrics
Instead of trying to force-fit AI into traditional frameworks, we should be asking: What new metrics can we create to truly capture AI's impact?
One obvious candidate is user satisfaction with AI-driven interactions. While MTTR focuses on speed, satisfaction measures the quality of the service. Did the user feel the AI was helpful? Was the issue resolved to their satisfaction? These are questions that could provide a more nuanced view of AI’s effectiveness.
Another important area to look at is how efficient the AI processes themselves are. This means looking at things like how many tasks the AI can handle on its own, how often it makes mistakes, and how quickly it can be trained to perform at its best. The focus isn't just on the final results, but also on the journey: How effectively is the AI learning? How quickly can it adapt to new and different requests?
By adopting these kinds of metrics, we get a much fuller picture of performance in an AI-driven world. We can better appreciate the value AI brings to the table while also recognizing where human expertise still plays a crucial role.
Developing and refining these measurements is essential to truly understand and improve the collaborative efforts of humans and AI working together to deliver outstanding service.
Benchmarking AI against AI, not against humans
It’s becoming increasingly clear that to truly understand and harness its potential, we must benchmark AI against AI, rather than against human performance. We're moving away from the old way of measuring technology by how well it could mimic or replace human tasks. Now, the questions are different: How does one AI system compare to another? What makes one stand out from the rest?
Benchmarking AI against AI takes us into new territory. The standards we use to evaluate these systems need to be just as cutting-edge as the technology itself. Traditional metrics like speed and accuracy are just the beginning. To really gauge an AI system’s effectiveness, we have to look deeper — at how well it juggles multiple tasks, how quickly it learns, and how capable it is of handling complex actions on its own.
With AI becoming an integral part of operations, choosing the right system can have a profound impact on efficiency, innovation, and competitive advantage. By benchmarking AI against AI, organizations can ensure they’re not just adopting the latest technology, but the right technology for their specific needs.
Let’s break these down.
Learning capabilities
Another critical aspect of benchmarking AI is its learning capability. AI systems are not static; they are designed to learn and improve over time.
But how quickly and effectively does an AI system learn? This involves assessing not just the volume of data the AI can process, but how efficiently it can translate that data into actionable insights or improved performance.
Does the AI system require frequent retraining, or can it adapt on the fly? How well does it learn from past mistakes to prevent future errors? The answers to these questions can reveal a lot about the long-term viability and scalability of an AI system.
Capacity for autonomous complex action
As AI evolves, its ability to perform increasingly complex tasks autonomously will be a critical benchmark. It’s one thing for AI to handle routine, straightforward tasks, but quite another to manage complex processes that require a deep understanding of context, interdependencies, and potential outcomes.
For example, consider an AI agent tasked with managing a database – identifying slow queries, diagnosing the underlying code issues, and implementing fixes, all without human intervention. This requires a sophisticated level of autonomy, where the AI not only follows a set of predefined rules but also makes informed decisions based on real-time data and context.
Handling multiple tasks simultaneously
One of the key advantages of AI is its potential to handle multiple tasks at once, far beyond the capabilities of a human operator. In benchmarking AI, it’s critical to assess how well a system can juggle various responsibilities in parallel.
For instance, can an AI agent simultaneously process a user’s request, analyze data patterns, and update system configurations without dropping the ball on any of these tasks? The ability to manage and prioritize multiple tasks in real-time is a major differentiator between AI systems and one that could define their value in complex environments.
As AI continues to advance, it’s essential to move beyond traditional comparisons with human performance and instead develop robust benchmarks that allow us to evaluate AI systems on their own terms. This approach will enable us to fully appreciate the capabilities of AI and harness its potential to drive innovation and success in ways we’re only beginning to understand.
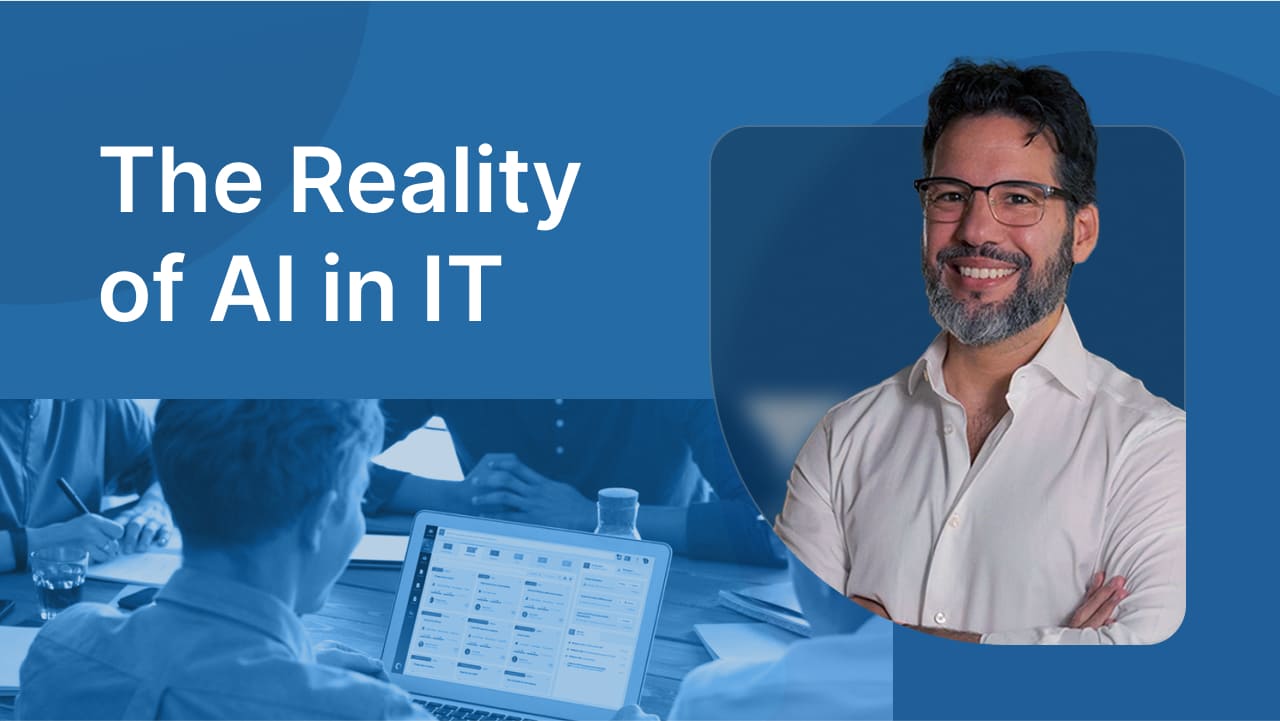
Bridging The Gap: The Marketing Hype vs. The Reality of AI in IT
The future of AI metrics
The capabilities of AI are evolving rapidly. I envision a future where AI agents could manage highly complex tasks, such as analyzing database performance, correcting code, running quality assurance tests, and even deploying updates – all autonomously. While we’re not quite there yet, the trajectory is clear: AI is becoming more capable every day.
Organizations need to prepare for this future by rethinking how they measure success. Instead of clinging to outdated metrics, we should start developing and implementing new ones that align with AI’s capabilities.
This might involve redefining what success looks like in an AI-driven world, focusing not just on speed and efficiency but also on accuracy, user satisfaction, and ethical considerations.
Conclusion
In short, the traditional metrics we’ve relied on for decades are becoming obsolete in the face of AI’s rapid advancement. As AI takes on more tasks and becomes an integral part of IT processes, we need to rethink how we measure success.
New metrics focused on user satisfaction, AI efficiency, and governance are essential for capturing the true value of AI. As we continue to explore these new possibilities, we need to open the discussion on how we can best measure success in this new landscape.
Let’s start reimagining our metrics today so we’re ready for the AI-driven future just around the corner.