Choosing the right platform to handle and analyze your company’s data is more critical than ever. Databricks has become a well-known player in the industry, offering a robust data intelligence platform that integrates data engineering, machine learning, and data science. However, for companies seeking alternatives, several Databricks competitors can meet varying needs and preferences, allowing you to choose the perfect fit.
Whether you’re exploring Databricks for the first time or considering a switch, we’ve compiled a list of powerful competitors in 2024. These platforms provide robust features for businesses of all sizes, ensuring you can find the one that aligns with your specific data needs and objectives.
Databricks Data Intelligence platform top 7 competitors
The landscape of data intelligence platforms has evolved rapidly, with many players offering unique features that distinguish them from Databricks. While Databricks has made a name for itself, exploring its competitors can provide new perspectives on functionality, scalability, and pricing, which are essential when making decisions for your business.
Choosing the right platform means evaluating various factors such as ease of use, compatibility with your existing systems, pricing models, and scalability. Below, we delve into seven top competitors that provide powerful alternatives to Databricks.
1. Snowflake
Snowflake has risen to prominence as a highly efficient, cloud-native data platform designed for storage, analytics, and data sharing. With its unique architecture that separates compute from storage, Snowflake provides the flexibility and scalability that businesses need in today's data-intensive environments. Its automatic scaling and multi-cloud support have made it a preferred choice for many organizations looking to modernize their data operations.
The platform allows businesses to process massive amounts of data across clouds, offering seamless integration with AWS, Azure, and Google Cloud. Snowflake's elastic performance allows companies to quickly scale up or down based on their processing needs, all while maintaining secure, efficient data management. This adaptability, combined with its user-friendly interface, has helped Snowflake carve out a substantial presence in the data intelligence space.
Pricing
Snowflake offers a usage-based pricing model, with separate pricing for storage and compute resources. There are many pricing options for Snowflake. This flexibility in pricing allows businesses to only pay for the resources they actually use, making it a cost-efficient solution for growing companies.
Pros
- Automatic scaling and separation of storage and compute.
- Broad support for data formats and sources.
- Multi-cloud deployment across AWS, Azure, and Google Cloud.
Cons
- Pricing can become expensive for high compute needs.
- Limited built-in machine learning features compared to Databricks.
Features
- Secure data sharing across different organizations.
- Time travel and cloning features for efficient data management.
- Support for semi-structured and structured data.
Google BigQuery
Google BigQuery is Google Cloud’s fully-managed, serverless data warehouse that is known for its lightning-fast analytics and real-time insights. Built with scalability in mind, BigQuery helps businesses analyze large datasets effortlessly by leveraging Google’s underlying infrastructure, which is highly performant and secure. With BigQuery, companies can focus on analyzing their data without needing to worry about managing infrastructure, making it an attractive option for enterprises focused on efficiency.
BigQuery stands out for its ability to handle data from various sources while ensuring seamless integration with Google Cloud services like Google Analytics, Google Sheets, and Looker. This tight integration with the Google Cloud ecosystem makes it a go-to choice for businesses already utilizing Google's services. Its advanced analytics capabilities, combined with the flexibility to run SQL queries on massive datasets, are some of the reasons BigQuery is a top competitor in the data intelligence space.
Pricing
Google BigQuery follows a flat-rate or on-demand pricing model. For more information, you will need to contact sales.
Pros
- Serverless architecture eliminates the need for infrastructure management.
- Seamless integration with other Google Cloud services.
- Real-time analytics capabilities.
Cons
- Limited support for non-Google cloud platforms.
- Pricing can increase rapidly with complex queries.
Features
- Built-in machine learning (ML) using BigQuery ML.
- Support for geospatial analysis.
- Ability to query data across multiple clouds without moving it.
Amazon Redshift
Amazon Redshift is a powerful, fully managed data warehouse service built by AWS to handle complex analytical queries and deliver fast performance. Known for its ability to scale efficiently, Redshift can handle petabytes of data, making it an ideal solution for businesses dealing with massive datasets. Redshift is designed to integrate seamlessly with other AWS services, such as Amazon S3 and Amazon EC2, giving it an edge for companies already operating within the AWS ecosystem.
In addition to its integration with AWS services, Redshift offers a columnar storage architecture that optimizes query performance and speeds up data retrieval. This makes it a strong competitor to Databricks, especially for businesses looking for a data warehouse that delivers on both speed and scalability. Redshift's extensive data-sharing capabilities and machine learning integration make it a suitable choice for enterprises seeking a reliable, cloud-native analytics solution.
Pricing
Amazon Redshift offers two pricing models: on-demand and reserved instances. On-demand pricing starts at $0.25 per hour for DC2. Large instances, while reserved instance pricing offers discounts based on the commitment term. Reserved instances can offer savings of up to 75% over time.
Pros
- Integration with the AWS ecosystem.
- Excellent performance for complex queries.
- Scalability for large datasets.
Cons
- Performance can degrade for small datasets.
- Complexity in setup and configuration for larger deployments.
Features
- Columnar data storage for efficiency.
- Support for large-scale data migration with AWS Data Migration Service.
- Automated backups and data encryption.
Azure Synapse Analytics
Azure Synapse Analytics is Microsoft’s unified platform for big data and data warehousing. It offers an integrated experience that combines data integration, data warehousing, and big data analytics, giving businesses a holistic approach to managing and analyzing their data. With its built-in capabilities for real-time analytics, Azure Synapse is an excellent option for companies looking for both operational and analytical insights from their data.
Azure Synapse Analytics' deep integration with Microsoft services such as Power BI, Azure Machine Learning, and Azure Data Lake enhances its appeal to organizations already invested in the Microsoft ecosystem. Its ability to process large datasets quickly while maintaining a flexible pricing model allows businesses of all sizes to harness the power of their data in a cost-effective manner.
Pricing
Azure Synapse Analytics pricing is divided into on-demand pricing for serverless queries at $5 per TB processed and provisioned resources starting at $1.20 per DWU (Data Warehousing Unit) per hour. Businesses can choose the pricing model that best suits their usage patterns.
Pros
- Seamless integration with Azure services and Power BI.
- Unified analytics experience for data warehousing and big data.
- Serverless options for cost control.
Cons
- Complex setup for non-Microsoft users.
- Costs can rise quickly with extensive usage.
Features
- Native machine learning capabilities.
- Real-time data processing with Apache Spark.
- Built-in data governance and security controls.
Dremio
Dremio positions itself as an open-source, high-performance data lake engine that allows organizations to perform fast, interactive queries directly on data lake storage. Dremio eliminates the need for ETL (Extract, Transform, Load) processes, enabling businesses to query data in place. This reduces the complexity of data movement and empowers users to analyze data more quickly and cost-effectively.
As a highly customizable platform, Dremio enables organizations to build their own data infrastructure that fits their unique needs. Its in-memory acceleration feature allows users to experience faster query performance on large datasets, making it a strong contender for companies that prioritize performance and flexibility in their data analytics solutions.
Pricing
Dremio's community edition is free and open-source, while the enterprise version starts at $12,000 per year, based on the number of nodes in the cluster. This makes Dremio accessible for smaller teams while offering scalable options for larger enterprises.
Pros
- Open-source and highly customizable.
- In-memory data acceleration for faster queries.
- Direct querying on data lakes without ETL processes.
Cons
- Limited built-in support for machine learning.
- Requires more manual configuration and optimization.
Features
- Apache Arrow for fast in-memory processing.
- Integration with BI tools like Tableau and Power BI.
- Support for both structured and unstructured data.
Cloudera
Cloudera provides a comprehensive enterprise data cloud platform that supports hybrid and multi-cloud environments. As an open-source solution, Cloudera offers a flexible approach to data management and analytics, making it a favorite among organizations that require robust data security and governance features. Cloudera’s ability to integrate with various cloud services and its extensive support for big data technologies make it a strong competitor in the data intelligence market.
Cloudera's strength lies in its ability to manage and analyze data across different environments, whether in the cloud, on-premise, or hybrid deployments. With advanced features for data security, governance, and machine learning, Cloudera is a reliable choice for businesses with complex data needs and a focus on compliance.
Pricing
Cloudera pricing follows a subscription-based model. Its structure allows Cloudera to serve businesses with varying needs, from small startups to large enterprises.
Pros
- Strong data governance and security features.
- Hybrid and multi-cloud support.
- Open-source flexibility.
Cons
- Pricing can be high for smaller teams.
- Steeper learning curve compared to fully managed solutions.
Features
- Extensive support for big data tools like Hadoop and Spark.
- Built-in machine learning and analytics.
- Data lineage tracking and auditing.
DataRobot
DataRobot is an AI-powered platform that focuses on automating the development of machine learning models. Known for its ease of use, DataRobot simplifies the model-building process, allowing data scientists and business users alike to develop accurate predictive models without extensive programming skills. This makes it an attractive choice for companies looking to accelerate their AI initiatives while maintaining strong model performance.
DataRobot’s automation extends to data preparation, feature engineering, and model deployment, making it a comprehensive solution for businesses that want to fast-track their AI projects. By democratizing access to AI tools, DataRobot has positioned itself as a top competitor to Databricks, particularly for organizations that prioritize machine learning over traditional data analytics.
Pricing
DataRobot’s pricing starts at $20,000 per year for its basic AI Cloud platform, with custom pricing available for enterprise-level features and deployments. This tiered pricing structure makes it accessible for businesses of different sizes.
Pros
- Automated machine learning model development.
- User-friendly interface for both data scientists and non-technical users.
- End-to-end AI lifecycle management.
Cons
- Higher pricing for advanced features.
- Limited data warehousing capabilities compared to other platforms.
Features
- Automated data preparation and feature engineering.
- Integration with cloud storage platforms like AWS, Azure, and Google Cloud.
- Comprehensive model evaluation and governance.
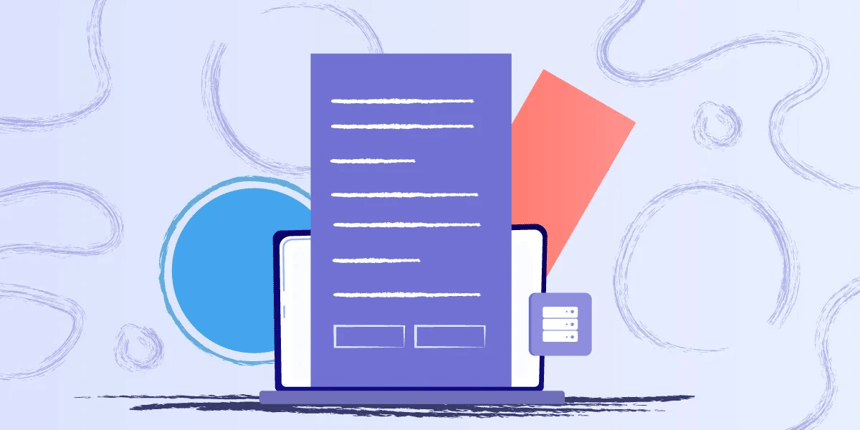
What is data analytics?
Data analytics refers to the process of examining raw data to find trends, draw conclusions, and help in decision-making. It has become a vital function for businesses aiming to make data-driven decisions. Through data analytics, organizations can improve operational efficiency, enhance customer experience, and identify new market opportunities.
Data analytics platforms like Databricks and its competitors provide the tools and technologies needed to perform these analyses at scale. Whether using machine learning models, statistical methods, or simple data queries, these platforms empower organizations to leverage data to their advantage.
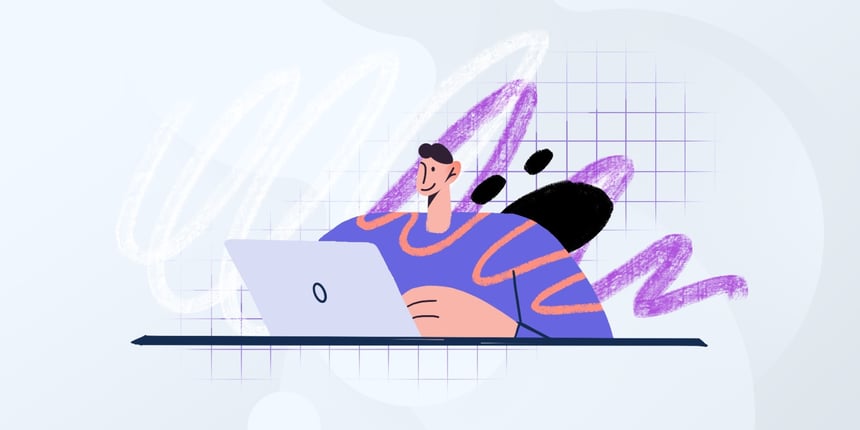
To sum up
The right data analytics platform depends heavily on your company’s specific needs. Databricks stands out for its seamless integration of data engineering and machine learning. However, other platforms, such as Snowflake, Google BigQuery, and Amazon Redshift, offer compelling alternatives with unique strengths.
By carefully evaluating features, pricing models, and integration capabilities, you can make an informed decision that will positively impact your data strategy. The competitors we’ve highlighted here present strong alternatives that could align better with your company’s goals and resources.
Frequently Asked Questions (FAQs)
1. What is the best alternative to Databricks?
It depends on your specific needs. Snowflake is an excellent choice for cloud storage and analytics, while Google BigQuery excels in serverless data warehousing.
2. Is Databricks suitable for small businesses?
Databricks can be scaled down, but its complexity and pricing may be more suitable for medium to large enterprises.
3. How does pricing compare between Databricks and its competitors?
Pricing varies significantly. For instance, Snowflake and Google BigQuery operate on usage-based models, while platforms like Cloudera and Teradata offer subscription-based pricing.
4. Can I migrate from Databricks to one of these competitors?
Yes, many of these platforms provide migration tools or services to help transition your data and workloads.